Dr Peter Krzystek – The Future of Forest Mapping
Having good knowledge of forest structures forms the basis for planning and management, and assessing the overall health of a woodland ecosystem. Over the past 15 years, automated remote sensing techniques for monitoring forests have become more widespread, allowing us to map much larger areas at finer scales. By combining laser scanning, aerial imagery and advanced computational techniques, Dr Peter Krzystek of the Munich University of Applied Sciences and his colleagues are leading the way in 3D forest mapping.
Covering approximately 31% of the world’s land surface, forest ecosystems are one of the most important living systems on Earth. They hold the vast majority of the world’s terrestrial species, provide oxygen and watershed protection, store carbon, prevent soil erosion, and mitigate climate change. Within these ecosystems are forest structures such as individual species, single trees and fallen dead trees.
Dead trees are of particular significance. Approximately one third of all animals and plants living in forests rely on dead wood. As dead wood is decomposed by fungi, bacteria and other life forms, it facilitates new plant growth, by recycling nutrients such as nitrate and phosphorus back into the ecosystem. Furthermore, recent studies have estimated that up to 11% of all greenhouse gas emissions are sequestered by the world’s forests, while 14% of total carbon stocks in forests are contained within dead wood.
Knowledge of dead wood, and forest structures in general, is fundamental to understanding, protecting and preserving the biodiversity of our forests. As well as gaining a good understanding of ecological health, comprehensive environmental monitoring of our forests and the structures within are also important in disaster management. From monitoring entire forests in the case of wildfires, to tracking the spread of disease in single and dead trees – gaining accurate information on the status and distribution of these structures over various time scales is vital. This information is used by forest managers, researchers and governmental and inter-governmental institutions.
In the past, we relied on conventional forest inventory to monitor forests – vertical-view aerial photography for imaging, field studies and manual methods of subdividing the forest area into certain grid sizes, and the forest into circular plots. This meant high costs and limited spatial coverage. In the 1970s, with the launch of satellite technology and the advent of remote sensing and digital mapping technology, the way forests were mapped was changed forever, making it possible to map at very large scales.
‘The innovative methods are also applicable to map urban objects (trees, cars, buildings, etc.) or just recently, vegetation and unknown graves of radioactive biomass in Chernobyl’s exclusion zone.’
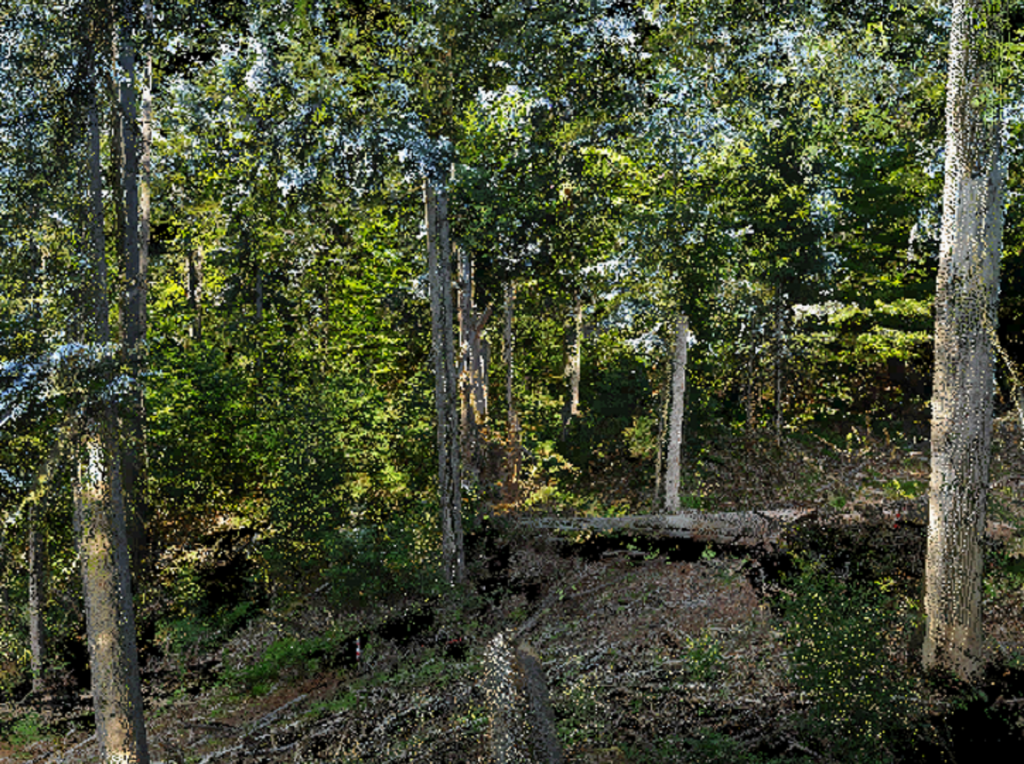
Fallen dead trees
Nowadays, a new technique called Light Detection and Ranging (LiDAR) is facilitating scientific discoveries at a phenomenal pace. LiDAR is a remote sensing technique where pulses of visible or near-infrared laser light at a particular wavelength are used to create 3D images. Pulses are first emitted towards an object, such as a forest, and the reflected pulses are detected using a sensor. The return times of the reflected laser light can then be used to obtain accurate distances between the different parts of the object and the sensor, allowing a 3D image to be built. This 3D image is typically made up of a high density of data points, known as ‘point clouds’.
LiDAR is also known as laser scanning, and has aerial, terrestrial and mobile versions. In the aerial version, the system is fixed onto an aircraft, helicopter or drone, and is capable of scanning large swathes of land. Terrestrial LiDAR, on the other hand, is a stationary system where the sensor is usually mounted on a tripod. Mobile LiDAR is also a ground-based system, but is typically mounted on a moving vehicle.
In their recent research, Dr Peter Krzystek and his team at the Munich University of Applied Sciences have focused their efforts on developing innovative methods using LiDAR and aerial imagery for 3D mapping of trees in the Bavarian National Forest in Germany.
Capturing Remote Sensing Data
Over a ten-year period, Dr Krzystek and his team conducted numerous flight campaigns in the Bavarian National Forest – an excellent test site for the research. The flying height of the operation was between 400 and 600 metres, with the LiDAR system emitting more than 400,000 laser light pulses per second.
The team’s LiDAR sensor recorded in ‘full-waveform’ mode. Full-waveform technology adds significant value to the dataset, because it records the entire time series of received light and receives many more reflected signals from each emitted light pulse. This means that the data can be interrogated for more information about the composition of the objects in question, resulting in more precise maps.
The team combined their LIDAR data with aerial images obtained using cameras with multispectral sensors. The images collected with these cameras are often used along with LiDAR to generate radiometric data, which offers additional information on the intensity of the reflections of the tree species. This information can aid in the classification of tree species and the identification of standing dead trees.
In conjunction with LiDAR and aerial imagery, Dr Krzystek and his team also applied a series of advanced computer techniques known as machine learning and computer vision. Machine learning is a technique used to teach computers how to think for themselves, and in this situation the computer was taught how to find tree objects such as crowns and stems. Computer vision, on the other hand, is a type of machine learning designed specifically to analyse imagery.
‘My main research focus is on the application of machine learning methods and computer vision to detect and classify forest objects – like single trees, fallen or standing dead wood,’ says Dr Krzystek. ‘The overall goal is to develop techniques for automatic forest inventory using remote sensing data (that is, laser scanning data or image data) acquired from airborne platforms like planes, helicopters or drones.’
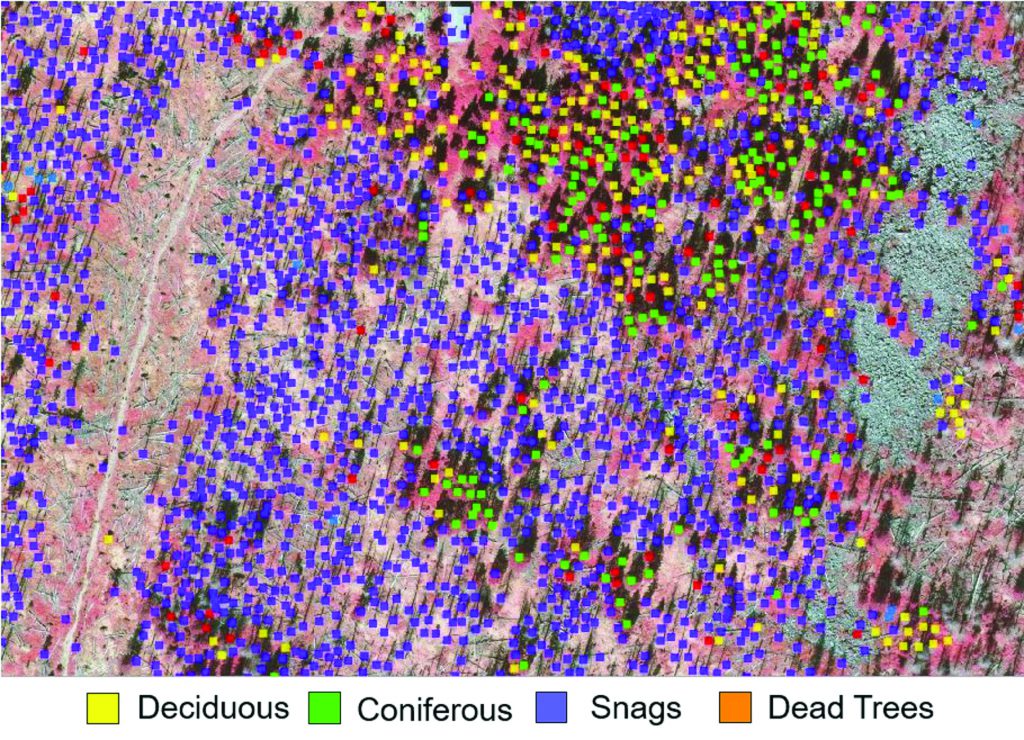
Mapping of coniferous, deciduous, and dead trees (Image source: Bavarian Forest National Park)
Detecting Forest Structures
The methods developed by Dr Krzystek and his team demonstrate that forest areas can be completely and automatically processed with LiDAR to produce 3D maps of individual trees, even at very large scales. ‘The results of our methods enable an area-wide 3D vegetation mapping and provide precise information about the percentage of tree species, stock of wood, wood growth, wood harvest in forests, and biomass,’ Dr Krzystek explains.
One of the team’s methods offers the ability to detect single trees, and was one of the breakthroughs of this project. The team’s newly patented approach takes advantage of a special segmentation technique adapted from image analysis. This technique involves labelling every point in the point cloud, and grouping these points. When combined with full waveform data, the detection rate is significantly improved. With this method, Dr Krzystek and his team found that they could classify coniferous and deciduous trees with excellent accuracy.
The team was then able to classify four tree species with fairly good accuracy by combining a large set of features generated from aerial imagery and multispectral LiDAR. Multispectral LiDAR acquires data at different wavelengths, and by using this, more data can be gained on the composition of the object, such as a single tree.
The team’s methods are also particularly good at detecting fallen and standing dead trees. Accuracy is strongly dependent on the number of points collected by aerial and terrestrial LiDAR, but other factors such as the density of the forest canopy, the length of the stem and numbers of trees are also important. In training the computers to recognise fallen trees, Dr Krzystek and his team observed that there must be a minimum number of collected data points per tree – otherwise, the computer can’t see the tree.
Using their new techniques, the research team found that they could also successfully detect standing trees with and without crowns – although trees without crowns were typically characterised using aerial LiDAR alone, as the trunks cannot be detected using aerial imagery alone. The team found that aerial LiDAR and aerial imagery perform equally well in the upper canopy, while aerial imagery performed less well in the medium to lower moving canopies.
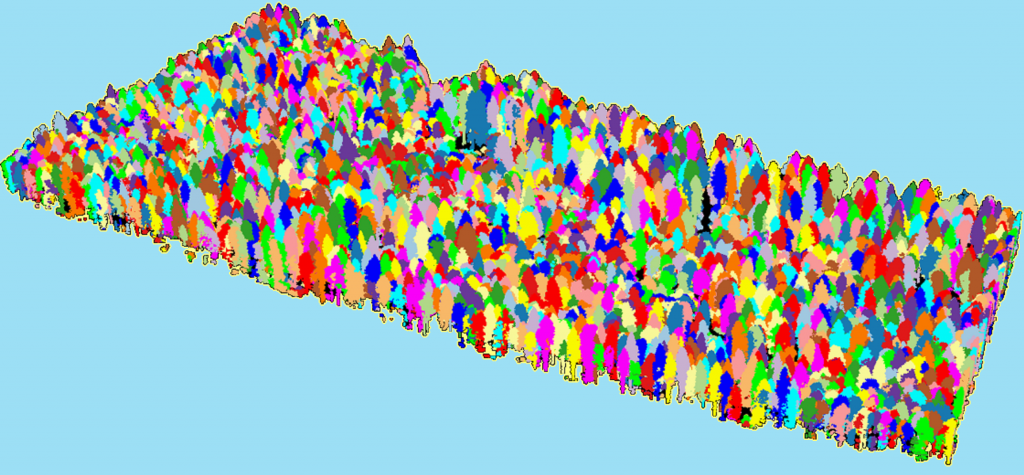
Segmented single trees
The Future of 3D Forest Mapping
Mapping is critical for forest inventory, conservation and planning, and LiDAR technology offers researchers the opportunity to gain much more data in a short space of time. Furthermore, the use of LiDAR is increasing and the cost is declining. LiDAR sensors are becoming more powerful, allowing even more data points to be obtained. ‘Since it is expected that new 3D measuring techniques will generate more 3D details of forest objects, existing methods need to be extended to take advantage of the higher point density,’ adds Dr Krzystek.
The team now hopes to evaluate their methods that characterise fallen trees by incorporating mobile LiDAR technology such as wearable scanners, and photogrammetric point clouds – sets of 3D data points produced from images as opposed to laser pulses. The team also hopes to investigate tree species classification further by using multispectral laser scanning data.
LiDAR-based 3D data has wide applications beyond forestry. Dr Krzystek and his team assert that the methods they developed to characterise fallen trees could potentially be applied to urban features, or groupings of pipelines and road networks.
The team’s methods have already been applied to hazard analysis for workers involved in remediation work: ‘The innovative methods are also applicable to mapping urban objects (trees, cars, buildings, etc.) or just recently, vegetation and unknown graves of radioactive biomass in Chernobyl’s exclusion zone,’ concludes Dr Krzystek.
Meet the researcher
Dr Peter Krzystek
Department of Geoinformatics
Munich University of Applied Sciences
Munich
Germany
Dr Peter Krzystek completed his PhD at the Institute of Applied Geodesy for Construction, University of Stuttgart in 1989. During this year, he moved to Inpho GmbH in Stuttgart for his second research assistant position in digital photogrammetry and software development. Dr Krzystek started his current position as Professor of Photogrammetry, Remote Sensing and Digital Image Processing at Munich University of Applied Sciences in 1998. Here, he specialises in a wide variety of research areas, including machine learning and computer vision, LiDAR-based 3D mapping and forest inventory. He is Head of the Photogrammetry and Remote Sensing Laboratory and has led the CORSNAV research group since 2012.
CONTACT
E: peter.krzystek@hm.edu
W: https://www.geo.hm.edu/kontakt/prof/krzystek/index.en.html
KEY COLLABORATORS
Bavarian Forest National Park
FUNDING
Bavarian State Ministry of Sciences, Research and the Arts
Federal Ministry of Education and Research
German Research Foundation
European Science Agency
FURTHER READING
S Briechle, A Sizov, O Tretyak, V Antropov, N Molitor, P Krzystek, UAV-based detection of unknown radioactive biomass deposits in Chernobyl’s exclusion zone, ISPRS TC II Mid-term Symposium, Towards Photogrammetry 2020, Riva del Garda, 04th – 07th June, 2018.
P Polewski, W Yao, M Heurich, P Krzystek, U Stilla, Detection of fallen trees in ALS point clouds using a normalized cut approach trained by simulation, ISPRS Journal for Photogrammetry and Remote Sensing, 2015, 105, 252–271.
J Reitberger, Cl Schnörr, P Krzystek, U Stilla, 3D Segmentation of single trees exploiting full waveform LIDAR data, ISPRS Journal of Photogrammetry and Remote Sensing, 2009, Vol. 64, No. 6, 561 – 574.