Professor René Laprise – Predicting Climate Change Impacts: Regional Climate Modelling Is A Critical Tool
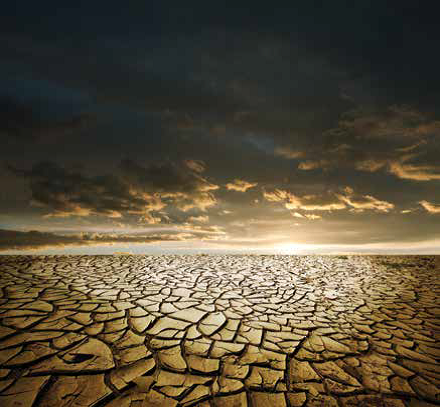
‘Given the quasi impossibility (and undesirability!) of experimenting with the real climate, models serve as a computational laboratory in which one can carry experiments to test hypotheses’
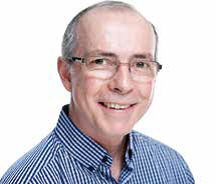
Global Climate Models (GCM)
A Global Climate Model (GCM) is software that uses a prescribed set of inputs (also called forcings) to predict future climate. Inputs may include, for example, greenhouse gas levels or land use changes such as deforestation or reforestation activities. The IPCC (Intergovernmental Panel on Climate Change) describes GCMs as ‘the most advanced tools currently available for simulating the response of the global climate system to increasing greenhouse gas concentrations’.
GCMs are computer programs based upon numerical models that take the geophysical properties that affect earth’s climate and use governing equations — known laws of physics — to determine exchanges of momentum, energy and mass between the atmosphere, hydrosphere and land surface. GCMs can be used to calculate changes over time for many variables, for example: pressure, humidity, precipitation, ocean salinity, sea ice, or snow cover, etc.
It’s a trade-off: model resolution versus computing power
GCMs apply a three-dimensional grid to the earth’s atmosphere and oceans. At each grid point, the supercomputer running the model solves the governing equations to provide data on the climate variables being simulated. The typical GCM consists of 30–60 vertical layers along its vertical axis and grid points 200 km apart on its horizontal axis in the atmosphere. In total, a GCM may consist of over a million of grid points across the full globe.
In addition to the spatial resolution, the temporal resolution (time-step) of GCMs also must be considered. A GCM with a temporal resolution of 30 minutes requires almost two million time-steps in a 100-year climate simulation. At each time-step, all model variables are calculated at each of the (millions of) grid points!
Increasing the resolution of a model involves either increasing computer processing speed or increasing the time it takes for completing a simulation. As Professor Laprise explains: ‘if a simulation took one week of computer time with a 200 km grid, it would take over two years of computations with a finer scale grid of 40 km – too long a waiting time for most!’ The average grid size of GCMs participating in century-long climate projections for the IPCC 5th Assessment Report was about 300 km. For perspective, at this scale, the UK would be covered by only about a half a dozen grid cells.
To keep within the bounds of current computing capabilities, and to reduce costs, climate modellers are forced to take some shortcuts. These include simplifying some fine-scale processes — for example, cumulonimbus cloud formation in thunderstorms, which, at an average of about 10 km in size, cannot be represented in a model with a resolution of 200–300 km. Although these fine-scale processes cannot be resolved by the model, they have an impact on the global climate and need to be accounted for, so scientists use parameterisation processes — mathematical equations that generalise the influence of these fine-scale processes on large-scale conditions in the model. As you might expect, the use of parameterisation processes can introduce substantial uncertainties into the model.
Computing advancements have enabled substantial increases in GCM resolution since the 1990s; but, the relatively coarse scale of GCMs continues to be a roadblock for climate modellers. Increases in the resolution of climate models are vital for predicting regional climate change impacts and for designing effective adaptation strategies for those consequences we can’t mitigate (for example, water resource management, or flood and coastal erosion prevention). The solution to this conflict between computing costs and the need for higher resolution data may lie in the use of Regional Climate Modelling.
Regional Climate Modelling
Regional Climate Modelling (RCM) has emerged over the past 20 years and allows researchers to remain within the bounds of affordable computing power while achieving, as Professor Laprise describes it, an ‘unprecedented amount of detail’ in climate simulations over sub-regions of the globe.
Reducing the area over which the model operates decreases computing cost and allows increasing spatial resolution. For example, EURO-CORDEX (the European arm of CORDEX, an international effort to produce improved regional climate change projections for all land regions worldwide) is working on grid cells of 12 km and making projections up to the year 2100. RCMs with grid sizes of 2.5–4 km are also being tested and models of this scale are able to reduce the simplification of convection, cloud and precipitation processes that can produce modelling uncertainty but is necessary in coarse-scale GCMs.
To make climate projections an RCM receives data from a GCM at its lateral boundaries (edges). In this way, the GCM provides starting inputs and driving information over time on large-scale variables (such as atmospheric temperature, winds and humidity), while the RCM provides increased resolution to calculate more precisely physical processes with improved representation of geophysical features within the study region — for example, mountain ranges and land use patterns. As a result, RCMs can inherit biases of the GCM that supplies data at its boundaries — for example, if the GCM is modelling a large-scale feature (such as El Niño – Southern Oscillation (ENSO)) poorly, then the RCM will inherit these biases and provide a poor simulation of regional ENSO effects. However, in cases where GCMs fail primarily due to coarse resolution of regional geophysical features and physical processes, RCMs are extremely helpful. For example, RCMs can be used to describe regional weather features such as lake-effect snow belts, regional monsoons, or the detailed precipitation patterns in regions with complex, fine-scale mountain ranges.
‘After working on the development of the first generation of Canadian Global Climate Model at the Meteorological Service of Canada, it seemed natural for me to move on to work on developing a high-definition (fine-mesh) Regional Climate Model that would allow resolving weather sequences with unprecedented amount of details’
Model Intercomparison Projects increase model validity
How are model results tested and validated? Professor Laprise says that, ‘while the main goal of climate models is to perform future climate-change projections, most of the efforts of climate modellers is dedicated to performing hindcast simulations for the recent-past climate and comparing these with available observational data to evaluate the skill (quality) of models’.
Because of the chaotic and complex nature of earth’s climate system, to identify statistically significant trends in climate modelling requires the use of model intercomparison projects, in which simulations from several models are pooled in order to produce mean values that outperform the ability of any single model.
An example of a model intercomparison project is CORDEX (COordinated Regional climate Downscaling EXperiment), an international coordinated effort to produce high-resolution climate change information derived from multiple models and suitable for impact and adaptation work. Professor Laprise says CORDEX and other model intercomparison projects are ‘most helpful to evaluate the skill of models and identify weaknesses that call for attention’.
Future research and influences on policy
Professor Laprise describes his current research as ‘strongly focussed on methodological aspects of Regional Climate Modelling’, including identifying the most optimal conditions for the application of RCMs with respect both to the size and the location of the study area, as well as the associated impact of the resolution jump from GCM to RCM at the edges of the RCM grid. His work aims to provide a set of operational rules that scientists can follow to achieve optimal application of RCMs and to reduce the uncertainties associated with the use of RCMs.
He and his colleagues are working to improve and validate several aspects of the fifth generation of the Canadian Regional Climate Model (CRCM5) and are also working on coupling the CRCM5 model with a regional ocean model such as NEMO (Nucleus for European Modelling of the Ocean).
In parallel to model improvement and applications, it is important to use detailed diagnostics to interpret the simulations and compare them to analyses of observations. With his team of students and research associates, Professor Laprise has developed recently a detailed energy budget formulation that allows evaluating the various energy transformations taking place in weather storms, and how these will change in a warmer climate.
Professor Laprise is one of the many scientists who volunteer their time and expertise as Lead Authors on assessment reports produced by the Intergovernmental Panel on Climate Change (IPCC). The IPCC is the international body for assessing the science related to climate change. IPCC assessments provide a scientific basis for governments at all levels to develop climate related policies, and they underlie negotiations at the UN Climate Conference – the United Nations Framework Convention on Climate Change (UNFCCC).
IPCC describes its assessments as ‘policy-relevant but not policyprescriptive’. The assessments present projections of future climate change based on different scenarios related to land-use change, population growth, energy use, etc., and discuss the risks that climate change poses as well as the social and environmental implications of different response options, but they do not tell policymakers what actions to take.
In 2007, the IPCC and former U.S. Vice-President, Al Gore, were jointly awarded the Nobel Peace Prize ‘for their efforts to build up and disseminate greater knowledge about man-made climate change, and to lay the foundations for the measures that are needed to counteract such change’.
Meet the researcher
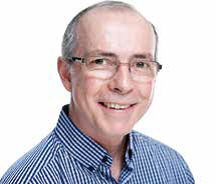
Professor René Laprise is widely recognised as the father of Regional Climate Modelling (RCM) in Canada. He has been a member of the faculty at Université du Québec à Montréal (UQAM), Montréal, Québec since 1988, and within the department of Earth and Atmospheric Sciences since 1995. Among his many accomplishments, is Professor Laprise’s contributions to the Intergovernmental Panel on Climate Change (IPCC) 4th Assessment Report (AR4) that was awarded the Nobel Peace Prize in 2007, ex aequo with former vice-president of USA, Al Gore. He served as Principal Investigator of the Canadian Network for Regional Climate Modelling for 15 years, and has received numerous honours for his contributions to the field. He has published over 129 peer-reviewed journal articles and 5 book chapters, and has trained 85 graduate students and 14 postdoctoral fellows during his tenure at UQAM.
CONTACT
E: laprise.rene@uqam.ca
W: http://professeurs.uqam.ca/professeur?c=laprise.rene
REFERENCES
L Hernández-Díaz, R Laprise, O Nikiéma, K Winger, 3-Step dynamical downscaling with empirical correction of sea-surface conditions: application to a CORDEX Africa simulation, Climate Dynamics, 2016, doi:10.1007/s00382-016-3201-9.
P Lucas-Picher, R Laprise, K Winger, Evidence of added value in North American regional climate model hindcast simulations using everincreasing horizontal resolutions, Climate Dynamics, 2016, doi:10.1007/ s00382-016-3227-z
M Clément, O Nikiéma and R Laprise, Limited-Area Atmospheric Energetics: Illustration on a Simulation of the CRCM5 over eastern North America for December 2004, Climate Dynamics, 2016, 22, http:// link.springer.com/article/10.1007/s00382-016-3198-0
D Matte, R Laprise, JM Thériault, Spatial spin-up of fine scales in a regional climate model simulation driven by low-resolution boundary conditions, Climate Dynamics, 2016, http://link.springer.com/ article/10.1007%2Fs00382-016-3358-2
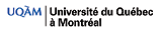