Dr Gregory Duane | Predicting Climate Change with Supermodels
Our universe is comprised of fascinatingly complex systems. Systems such as the Earth’s climate can, at first glance, seem far too complex and chaotic to predict accurately. Dr Gregory Duane and his team at the University of Colorado have been developing complex computational models that can learn from past data, providing us with intriguing insights and more accurate predictions about the future.
Predicting a Chaotic Universe
The universe around us is the product of interacting physical laws. By understanding and applying these laws, scientists can create models that simulate and explain the behaviour of the systems comprising the world around us. These laws can be used to describe the motions of simple systems (e.g., a pendulum) all the way up to hugely complex ones, such as the behaviour of the atmosphere and oceans driven by the different heating effects of the sun at the equator vs the poles.
One complication in all of this is chaos. In chaotic systems, the interplay between interactions gives outcomes that are impossible to predict. A good example of a chaotic system is the climate, neatly described by the butterfly effect. A butterfly flapping its wings can cause tiny changes in the air currents around it, and the ripple effect of these tiny changes in the air around the butterfly can lead to a hurricane developing halfway around the world. Of course, this outcome is very unlikely, but it is, nonetheless, technically possible. For simple systems, the final state can be easily predicted from the initial state, but for complicated, chaotic systems, the tiniest change in starting conditions could cause a completely different outcome. While the overall system may appear random from an outside perspective, this is not necessarily the case: if every interaction in a system can be understood, then it is theoretically possible to predict the outcome. In practice, however, predictions for chaotic systems are infeasible.
To properly model a system such as the Earth’s climate, scientists need to simulate each subsystem that comprises it, the interactions between these subsystems, the subsystems of the subsystems, and so on. This sort of simulation requires a phenomenal amount of computing power – an amount exceeding even the most state-of-the-art computers we have today. To work around this complexity, researchers make approximations about the smaller interactions; this allows the simulation to be run but also means they don’t paint the whole picture. Additionally, each research group will use different approximations, and thus, each simulation will tell a slightly different – and incomplete – story.
Developments in technology are allowing scientists to build the tools needed to achieve better predictions of chaotic systems. Dr Gregory Duane and his team from the University of Colorado have been investigating how we can optimise these simulations using innovative new techniques to produce systems that can predict real-world phenomena.
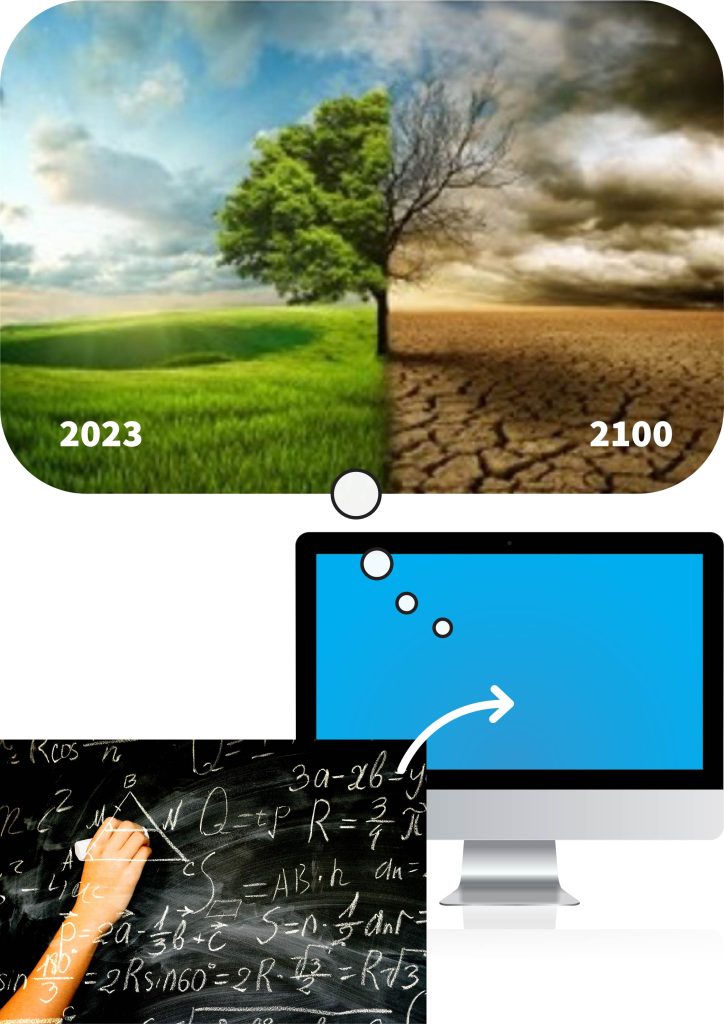
State-of-the-art computers use detailed climate physics to predict long-term climate change
Creating Accurate Climate Change Models
The most common use of climate models is to predict the weather. To do this, a computer ‘feeds’ available data received from weather instruments at regular time intervals into the simulation. This simulation will then use these data and physical laws to calculate what the weather is likely to be 30 minutes later. For example, given data about wind speed, direction, and local temperature, a calculation (prediction) can be made about the temperature downwind later in time. On larger scales, data about heating from the sun at the poles and equator can be used with an understanding of how hot and cold liquids rise and sink to calculate ocean currents, taking into account the force exerted on the ocean by surface winds and the deflection of movements of air or water due to the Coriolis effect from the Earth’s rotation. Ocean currents affect the heating of the atmosphere, which is involved in the calculation of wind speed and direction at a later time.
If no new data are available 30 minutes later, the process is simply repeated using the data predicted by the simulation. As the climate is a chaotic system, the further in advance researchers try to predict the exact weather, the less reliable the prediction is. Current weather models are able to predict specific weather events fairly accurately up to 10 days in advance. If we run the simulation for 100 years in the future, rather than 10 days, researchers can build a ‘broad-strokes’ prediction of climate change, taking into account all future states of the simulation to give trends in global temperatures, rainfall, and sea levels.
Climate change is, without a doubt, one of the biggest issues confronting current and future generations. Sadly, increased human activity, pollution and emission of greenhouse gasses have led us to a position where we must take action to prevent a worst-case scenario. To formulate the best response, we need to understand how, where, and when the effects of climate change will occur.
To achieve a better understanding of climate change, we need to improve our long-term forecasting capability. Whilst long-term trends in average global temperature are roughly predictable, more detailed climate change predictions are more difficult. Modern climate models are limited in the amount they can process – even with state-of-the-art computers. Models divide the Earth into a three-dimensional grid of simulation points, which are placed along every line of latitude and longitude and at more than 100 vertical levels of the atmosphere and ocean. The models assign a temperature, pressure, wind speed, and so on, to each point, which are then used to compute the same quantities at a later instant of time. The model thus offers a full simulation of the three-dimensional state of the atmosphere and oceans. Part of the uncertainty surrounding the accuracy of these simulations arises from phenomena that are smaller than the area between simulation points on the grid, such as clouds.
Whilst these processes are very important to climate processes, simulations cannot compute them completely and instead make assumptions about how they affect the overall result, a method called ‘subgrid scale parametrisation’. Simulations in different models will use different assumptions, and this leads to variations in the results between these climate models. Dr Duane and his team wanted to not only improve predictions but entirely alter the way we approach this challenge by combining the different models.
The researchers specifically looked into the models’ simulation of extreme precipitation to predict situations where there will be unusually large or small amounts of rainfall, snow, and so on. This is essential because these factors can lead to some of the worst effects of climate change, including flooding and severe storms. Being able to predict the frequency of such events in different regions would help proactive efforts to protect people before extreme weather occurs. While current models already make such predictions, Dr Duane had an exciting insight into a way that they could be combined and improved – adapting them to past data in a unique way.
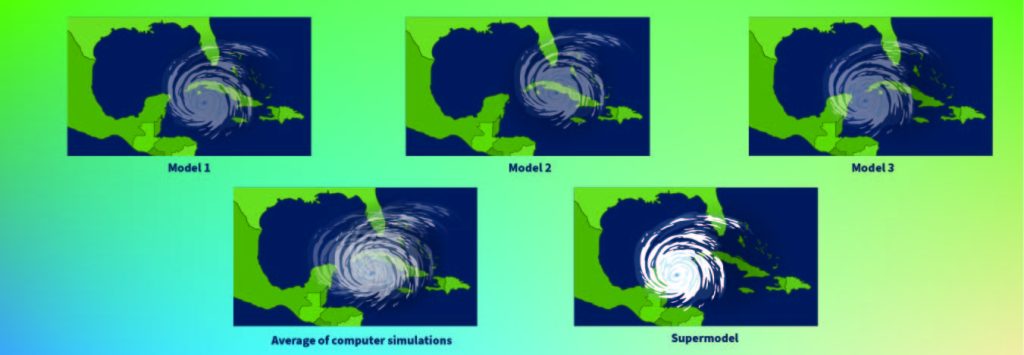
Hurricane simulations would synchronise in a supermodel, producing a sharper picture of the hurricane than would an average of three different computer simulations.
Synchronised Chaos
Of course, chaotic systems as a whole are naturally difficult to understand and predict. However, there is another natural phenomenon that can help make this job easier, and this is synchronisation. Synchronisation is the effect where multiple systems, which appear to be distinct from each other, naturally progress to the point of being ‘in sync’. This can be seen in nature, such as when pendulum clocks placed in close proximity begin to adapt and swing in time, the way the complex mechanisms in our body can adapt to our own circadian rhythm or the mysterious synchronisation of the hormonal cycles of female housemates. While it may seem almost supernatural, it is far from it, and by understanding the intricacies of the systems where it occurs, we begin to understand how synchronisation can be easily made to occur in the systems we construct. Surprisingly, even chaotic systems, though individually unpredictable, can synchronise with one another.
Using our understanding of synchronisation between chaotic systems, we can build a simulation of a chaotic system that synchronises with the real system. Weather models aim to take on information about the state of the real system and to duplicate the characteristics of the real system – synchronising the simulations with reality. Even with limited data, a simulation is able to synchronise with reality. Then, it is possible for the simulation to make predictions of the trajectory of the real system in the future. The details of the model software that performs the simulations must be adapted based on a repository of real data to augment the general physical laws – a process known as ‘training’. After training a model with 20th-century data, we can use it to predict the climate in the remainder of the 21st century, for which, of course, no data are available.
This has been the standard approach to weather and climate prediction, but the results for climate are unfortunately lacking. Each model is limited by the information it is provided and the way it utilises it, so the picture it creates of the real system may not be accurate. Different models, developed in different laboratories throughout the world, may use the same information in different ways and come up with different predictions. The simulations do not communicate with one another, and there is no natural way of combining the different predictions to form a unified result. That is, until Dr Duane and his colleagues put forward the solution of creating a new type of model that could fully take advantage of synchronisation to be induced between the different simulations: the supermodel.
Creating Supermodels
Historically, there have been many types of models for predicting the climate, differing especially in their parameterisations of small-scale processes, leading to conclusions that differ in important details, such as extreme precipitation. Generally, it was accepted that the best we could do was take the outputs from these models and average them to create a realistic guess as to how the climate will change over time.
Dr Duane had the innovative idea of creating a ‘supermodel’: instead of nudging a single simulation with data from the real world, his idea was to nudge any two simulations from different models toward each other. The two simulations feed information to each other, causing them to develop in time so that they become synchronised (or approximately so). As with the weather models, even a small amount of connectivity between simulations can create significant synchronisation between them.
To create an effective supermodel, Dr Duane and his team suggested the use of a diverse set of existing models that could potentially benefit from sharing capabilities with each other. Instead of training the models from scratch, starting from only general physical laws, this approach uses the collective wisdom of modellers in different centres who have made different choices (and arguably equally valid choices) about the difficult issues in modelling. Weights attached to variables determine their influence in the different models as they nudge each other. These weights are trained. This process allows the simulations to cooperate in real time with each other and produce a single synchronised result.
Dr Duane has been investigating how complex models, such as the ones used to predict climate change and extreme weather events, can be improved by exploiting synchronisation between different models describing the same process. Interactions in the atmosphere and oceans can cause a pattern to be present in the system: a coherent structure such as a front (warm or cold), a jet stream, or a hurricane that can move from place to place but maintains its essential form. In a supermodel, the coherent structures produced by different models would synchronise with each other and the real system.
This approach promises much better predictions of extreme weather events, such as storms that arise from coherent structures in the atmosphere. Unlike the traditional weighted averaging of simulations, the synchronised supermodel is much better at representing consistent atmospheric structures such as the jet stream. In the supermodel, these structures form in the same location at the same time, whereas in conventional methods, these structures can be placed differently in different models; the impacts they have on the climate are averaged out and lost. This is key for climate prediction as it helps to produce a more realistic picture of what can happen if the structures change in frequency or position in the future.
Recently, Dr. Duane and his colleagues were able to create a supermodel that performed far more effectively than any one individual model that they tested. This approach can even work for simulations exhibiting unusual or erroneous behaviour; coupling them creates a supermodel which is effective overall. Surprisingly, the individual simulations can both exhibit the same errors, yet the supermodel formed by combining them still produces a superior result instead of exacerbating the issue. This research marks the groundwork for future supermodels to be created based on a larger set of existing expert models, which will revolutionise the way we go about predicting climate and other complex systems.
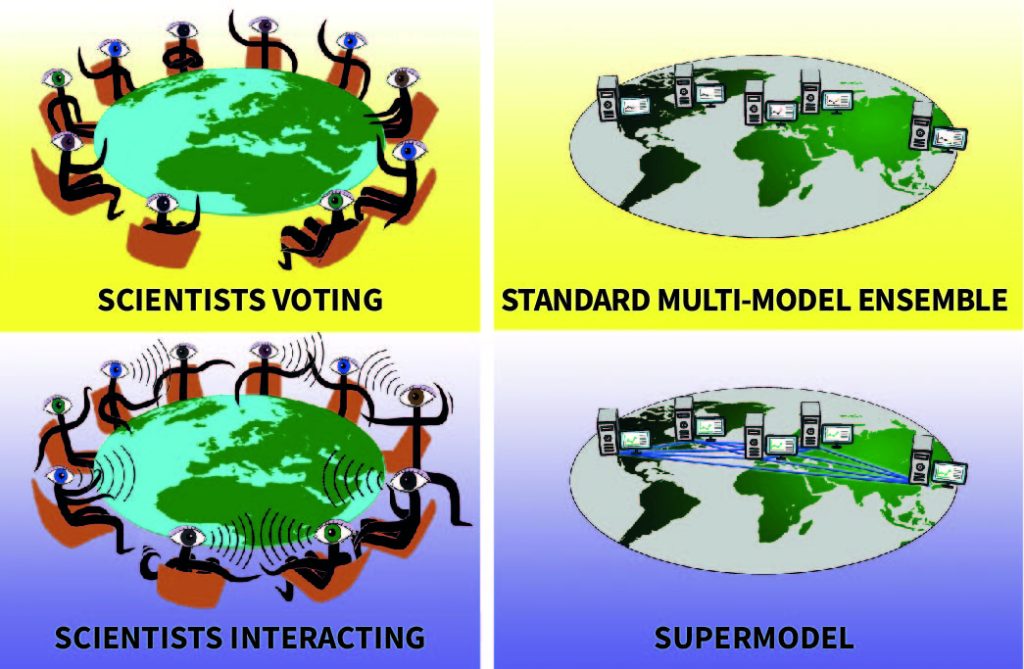
By interacting as they run, different computers produce a better result than obtained by combining their outputs – just as a group of scientists work better through discussion rather than simply voting or averaging their opinions.
Art in the left panels created by Vasil Chulev
Supermodelling the World
Dr Duane has shown that synchronisation is a powerful resource with which to better understand the seemingly unfathomable chaotic systems that surround us. Synchronisation is, in fact, so ubiquitous as to concur with the philosophical suggestion that there is an organisational principle of ‘synchronicity’ that rivals causality in importance.
These advances in the fields of climate modelling, chaos, and synchronisation allow better predictions of our future climate and a better understanding of how changes, large and small, will impact the complex climate system.
As supermodels can be utilised wherever we have many competing models for the same system, the approach is already being applied beyond climate science. For example, a supermodel was recently used to model the development of cancer tissue. Supermodelling could even be applied to unpick the workings of a powerful natural computer – the human mind. Dr Duane speculates that the conscious human mind may be regarded as the ultimate supermodel – observing itself at the same time as it observes reality – providing further justification for the use of this method with computers. Certainly, the potential of supermodelling to advance our understanding of the world is exciting, vast, and transformative.
SHARE
DOWNLOAD E-BOOK
REFERENCE
https://doi.org/10.33548/SCIENTIA969
MEET THE RESEARCHERS
Dr Gregory S Duane
Department of Atmospheric and Oceanic Sciences
University of Colorado Boulder
Boulder, CO
USA
In 1997, Dr Gregory Duane completed his PhD in astrophysical, planetary and atmospheric sciences at the University of Colorado, following undergraduate degrees in physics, math, and chemistry attained earlier at the Massachusetts Institute of Technology. After completing research and teaching posts at different institutions in the USA and further afield, and also spending time in industry, he was appointed to his current position as Principal Investigator on a National Science Foundation-funded project to develop supermodeling at the University of Colorado. He has concurrently held research positions at the Geophysical Institute at the University of Bergen (Norway), the Macedonian Academy of Sciences and Art (Macedonia), the Rosenstiel School of Marine and Atmospheric Sciences at the University of Miami (USA), the National Center for Atmospheric Research (USA) and The Institute of Mathematics and its Applications (USA). A consistent theme throughout his career has been the ubiquity of synchronisation in the climate system and elsewhere, and driving forward the supermodel programme to model climate more effectively.
CONTACT
E: gregory.duane@colorado.edu
W: https://www.colorado.edu/atoc/gregory-duane
KEY COLLABORATORS
University of Colorado Boulder, USA
Dr Francine Schevenhoven
Professor Jeffrey B Weiss
University of Bergen, Norway
Professor Asgeir Sorteberg
Professor Noel Keenlyside
Dr Mao-Lin Shen
Nansen Environmental and Remote Sensing Center, Norway
Dr Francois Counillon
Max Planck Institute, Hamburg, Germany
Dr Sebastian Rast, Dr Diego Jimenez
National Center for Atmospheric Research, USA
Dr Judith Berner, Dr William E Chapman
Royal Netherlands Meteorological Institute, Utrecht, Netherlands
Dr Frank Selten
Ecole Normale Superieure, Paris and UCLA, Los Angeles, California, USA
Professor Michael Ghil
FUNDING
National Science Foundation (Grant 2015618)
FURTHER READING
GS Duane, M-L Shen, Synchronization of Alternative Models in a Supermodel and the Learning of Critical Behavior, Journal of the Atmospheric Sciences, 2023, 80(6), 1565–1584. DOI: https://doi.org/10.1175/JAS-D-22-0113.1
F Schevenhoven, N Keenlyside, F Counillon, et al., Supermodeling: Improving Predictions with an Ensemble of Interacting Models, Bulletin of the American Meteorological Society, 2023, 104(9), E1670–E1686. DOI: https://doi.org/10.1175/BAMS-D-22-0070.1
GS Duane, C Grabow, F Selten, M Ghil, Introduction to focus issue: Synchronization in large networks and continuous media—data, models, and supermodels, Chaos, 2017, 27(12), 126601. DOI: https://doi.org/10.1063/1.5018728
GS Duane, Synchronicity from Synchronized Chaos, Entropy, 2015, 17(4), 1701–1733. DOI: https://doi.org/10.3390/e17041701
REPUBLISH OUR ARTICLES
We encourage all formats of sharing and republishing of our articles. Whether you want to host on your website, publication or blog, we welcome this. Find out more
Creative Commons Licence (CC BY 4.0)
This work is licensed under a Creative Commons Attribution 4.0 International License.
What does this mean?
Share: You can copy and redistribute the material in any medium or format
Adapt: You can change, and build upon the material for any purpose, even commercially.
Credit: You must give appropriate credit, provide a link to the license, and indicate if changes were made.
SUBSCRIBE NOW
Follow Us
MORE ARTICLES YOU MAY LIKE
Dr Robert Larkin | Cultivating Change to Improve Soil Health and Increase Potato Yield
Environmental quality and food production are facing the pressing challenges of climate change and global population growth. Dr Robert Larkin from the United States Department of Agriculture-Agricultural Research Service (USDA-ARS) and a team of plant scientists developed and tested a range of crop management systems to help overcome these compounding challenges. Their work is improving soil health and increasing the yield of potato crops, contributing to the future food security of nations.
Dr Olalla Castro-Alvaredo | Measuring Entanglement: Symmetry-Resolved Entropy
Dr Olalla Castro-Alvaredo of the City University of London (UK) and her collaborators are advancing our understanding of an important phenomenon of quantum mechanical systems known as entanglement and, especially, its mathematical measures. Symmetry-resolved entanglement entropy is one such measure. Their study focuses on special quantum states which are excited with respect to a ground state. The research shows how the entanglement amongst quantum particles can be measured and assesses the contribution to the entanglement of quasiparticle excitations, particularly in the presence of additional symmetries.
Dr Arne Stensvand | Thermotherapy: Effective Disease and Pest Management Without Chemicals
Dr Arne Stensvand and his team at the Norwegian Institute of Bioeconomy Research are developing physical methods of pest reduction in plants. The team is specifically interested in strawberry plants, for which pest management is vital for crop success. They are pioneering thermotherapy as a heat treatment method to provide an environmentally effective and economically sound non-chemical approach to pest management.
Dr. Daniel Canfield | Reprioritising Pollution Reduction in Florida’s Lakes
Florida’s landscape is dotted with thousands of lakes that reflect regional geology, topography and anthropogenic activities. Phosphorus and nitrogen are critical nutrients for maintaining the wide range of biological production expressed across Florida, but excessive inputs of these nutrients due to past human activities impair many waters. There has been a long history of work aiming to address associated water quality pressures, and Dr. Daniel Canfield at the University of Florida has been at the centre of these efforts for over 40 years. Now, with the correction of point-source nutrient inputs, Dr. Canfield proposes that holistic lake management, including the integration of in-lake management strategies with a focus on organic sediment removal, should be much more prominent on the US government’s agenda to provide faster restoration of stakeholders’ lake usability.